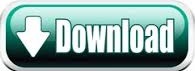
Although using a reply corrector allows one to assess the caliber of the corrections provided qualitatively, training the corrector has the disadvantage of being time-consuming. Due to its repetitive nature, its training and assessment loop might be extensive. JUICER has some drawbacks, even though it incorporates human feedback to enhance the effectiveness of dialogue agents.
JUICER demonstrated its capacity to use both positive and negative human feedback to enhance dialogue model performance by increasing good responses from 33.2 to 41.9 percent in the experiments and the F1 accuracy score from 15.3 to 18.5 on an unseen test set. The team’s method was applied to the base 3B parameter BlenderBot2 (BB2 3B) dialogue model on the FITS and DEMO datasets for their empirical study. The revised feedback and predictions from the earlier processes are then used to retrain the final dialogue model. The wrong responses are then changed into positive responses by the reply corrector.

The satisfaction classifier is trained to predict binary satisfaction labels and recognize positive and negative feedback for all unannotated bot responses. The suggested JUICER model comprises three primary modules: the dialogue model, a satisfaction classifier, and a reply corrector. The team’s top models ultimately perform better than the fundamental BlenderBot 2 model or Director alone. Models like Director (which uses both gold/predicted good and negative responses) further enhanced the final dialogue model. The research team also concluded that training with current gold corrections alone could perform better than training with corrections generated by reply-correctors. Training a satisfaction classifier to identify the unlabeled data and a reply corrector to map the poor replies to excellent ones extends sparse binary feedback. This system successfully applies binary and textual human feedback to enhance dialogue model conversational answers. In the recently published study: ‘When Life Gives You Lemons, Make Cherryade: Converting Feedback from Bad Responses into Good Labels,’ a research team from Meta AI and Columbia University suggests a framework known as JUICER as a step in this approach. However, humans cannot always give transparent or trustworthy cues indicating the same when a chatbot makes errors during encounters.

Although rare, human feedback in the wild usually takes the form of up or down votes, unstructured text comments, and “gold” edits. Deployed dialogue agents may use user feedback to enhance their performance over time. The machine learning community has recently concentrated a lot of its research on the best ways to leverage human feedback to enhance the performance and answers of chatbots and other language dialogue models.
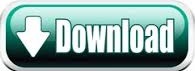